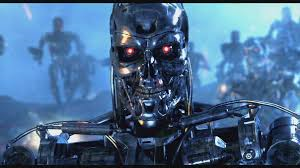
The mind-blowing AI announcement from Google that you probably missed.
…And then in September Google gave their translation tool a new engine: the Google Neural Machine Translation system (GNMT). The new engine comes fully loaded with all the hot 2016 buzzwords, like neural network and machine learning. The short version is that Google Translate got smart. It developed the ability to learn from the people who used it. It learnt how to make educated guesses about the content, tone and meaning of phrases based on the context of other words and phrases around them. And — here’s the bit that should make your brain explode — it got creative.
Google Translate invented its own language to help it translate more effectively From <https://medium.com/@GilFewster/the-mind-blowing-ai-announcement-from-google-that-you-probably-missed-2ffd31334805
Holy.... Our language is the encoding of reality...
https://alsionsbells.wordpress.com/2016/11/17/language-is-the-metaphor-for-time-change-the-language-change-time/ So sky net may not have to do any boots on the ground to accomplish what it did in the movie, all it would have to do is subtly change our language to create reality structure that we would be trapped in, if that isn't the case already.
Which IMO, already has. Note this one reply to the above article by a very intelligent human, on the subject, and that he at the very end says exactly that - The robots have already taken over 😉
You requested someone with a degree in this? *Holds up hand*
So there are two main schools of Artificial Intelligence — Symbolic and non-symbolic.
Symbolic says the best way to make AI is to make an expert AI — e.g. if you want a doctor AI, you feed it medical text books and it answers questions by looking it up in the text book.
Non-symbolic says the best way to make AI is to decide that computers are better at understanding in computer, so give the information to the AI and let it turn that in to something it understands.
As a bit of an apt aside — consider the Chinese room thought experiment. Imagine you put someone in a room with shelves full of books. The books are filled with symbols and look up tables and the person inside is told “You will be given a sheet of paper with symbols on. Use the books in the room to look up the symbols to write in reply.” Then a person outside the room posts messages in to the room in Mandarin and gets messages back in Mandarin. The person inside the room doesn’t understand Mandarin, the knowledge is all in the books, but to the person outside the room it looks like they understand Mandarin.
That is how symbolic AI works. It has no innate knowledge of the subject mater, it just follows instructions. Even if some if those instructions are to update the books.
Non-symbolic AI says that it’d be better if the AI wrote the books itself. So looking back at the Chinese Room, this is like teaching the person in the room Mandarin, and the books are their study notes. The trouble is, teaching someone Mandarin takes time and effort as we’re starting with a blank slate here.
But consider that it takes decades to teach a child their first language, yet it takes only a little more effort to teach them a second language. So back to the AI — once we teach it one language, we want it to be like the child. We want it to be easy for it to learn a second language.
This is where Artificial Neural Networks come in. These are our blank slate children. They’re made up of three parts: Inputs, neurones, outputs. The neurones are where the magic happens — they’re modelled on brains. They’re a blob of neurones that can connect up to one another or cut links so they can join one bit of the brain up to another and let a signal go from one place to another. This is what joins the input up to the output. And in the pavlovian way, when something good happens, the brain remembers by strengthening the link between neurones. But just like a baby, these start out pretty much random so all you get out is baby babble. But we don’t want baby babble, we have to teach it how to get from dog to chien, not dog to goobababaa.
When teaching the ANN, you give it an input, and if the output is wrong, give it a tap on the nose and the neurones remember “whatever we just did was wrong, don’t do it again” by decreasing the value it has on the links between the neurones that led to the wrong answer and of it gets it right, give it a rub on the head and it does the opposite, it increases the numbers, meaning it’ll be more likely to take that path next time. This means that over time, it’ll join up the input Dog to the output Chien.
So how does this explain the article?
Well. ANNs work in both directions, we can give it outputs and it’ll give us back inputs by following the path of neurones back in the opposite direction. So by teaching it Dog means Chien, it also knows Chien could mean Dog. That also means we can teach it that Perro means Dog when we’re speaking Spanish. So when we teach it, the fastest way for it to go from Perro to Dog is to follow the same path that took Chien to Dog. Meaning over time it will pull the neurones linking Chien and Dog closer to Perro as well, which links Perro to Chien as well.
This three way link in the middle of Perro, Dog and Chien is the language the google AI is creating for itself.
Backing up a bit to our imaginary child learning a new language, when they learn their first language (e.g. English), they don’t write an English dictionary in their head, they hear the words and map them to an idea that the words represent. This is why people frequently misquote films, they remember what the quote meant, not what the words were. So when the child learns a second language, they hear Chien as being French, but map it to the idea of dog. Then when they hear Perro they hear it as Spanish but map that to the idea of dog too. This means the child only has to learn about the idea of a dog once, but can then link that idea up to many languages or synonyms for dog. And this is what the Google AI is doing. Instead of thinking if dog=chien, and chien=perro, perro must = dog, it thinks dog=0x3b chien =0x3b perro=0x3b. Where 0x3b is the idea of dog, meaning it can then turn 0x3b in to whichever language you ask for.
Tl;Dr: It wasn’t big news because Artificial Neural Networks have been doing this since they were invented in the 40s. And the entire non-symbolic branch of AI is all about having computers invent their own language to understand and learn things.
P.S. It really is smart enough to warrant that excitement! Most people have no idea how much they rely on AI. From the relatively simple AI that runs their washing machine, to the AI that reads the address hand written on mail and then figures out the best way to deliver it. These are real everyday machines making decisions for us. Even your computer mouse has AI in it to determine what you wanted to point at rather than what you actually pointed at (on a 1080p screen, there are 2 million points you could click on, it’s not by accident that it’s pretty easy to pick the correct one). Mobile phones constantly run AI to decide which phone tower to connect to, while the backbone of the internet is a huge interconnected AI deciding the fastest way to get data from one computer to another. Thinking, decision making AI is in our hands, beneath our feet, in our cars and almost every electronic device we have.
The robots have already taken over 😉
Skynet... like a whole bunch of other stuff, happened while we were a sleep and distracted - in some other reality, in a galaxy far, far, away...
Wilcock and Corey Goode said that the biblical devil is an AI.